From AlphaGo to Machine Translation
- Miley Thomas
- April 5, 2016
- 4,438 views
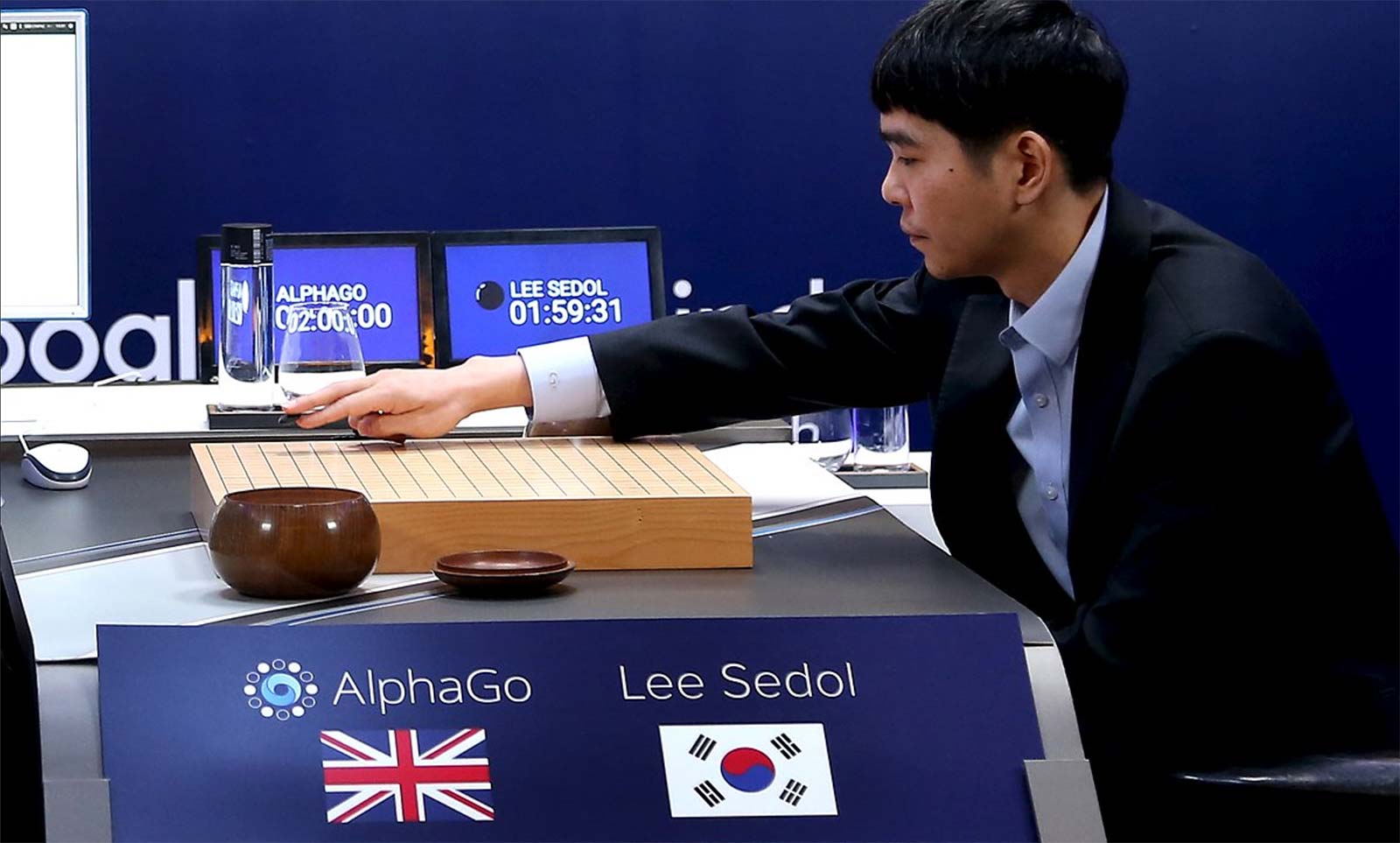
Lee Sedol had lost.
In a much-anticipated man-machine battle, the Google DeepMind development team’s AlphaGo defeated the South Korean champion of Go in the first three matches of a five match series. However, to a collective sigh of relief from spectators, Lee Sedol won the fourth game by exploiting flaws of AlphaGo, a dramatic stand for the human side. Unfortunately, he eventually lost the fifth match, meaning AlphaGo had still beaten the number three seed in the world’s Go playing masters.
For those not familiar with the game, Go is an ancient Chinese pastime that consists of a square board with 19×19 grid and identical black and white stone pieces. Two players place the stone pieces alternatively to take up vacant intersections, with the goal being to capture the largest area of the board. When an opponent’s pieces in an area are completely surrounded by your stone pieces with less than two empty spaces in between, you have “captured” all the enclosed pieces and take over that territory. Compared to International Chess, Go has many more pieces in play, thus creating greatly many more possibilities. When it comes to machine learning and processing of information, Go is exponentially more difficult for a computer to master than the Chess.
Artificial intelligence (AI) has come a long way since its first days. Twenty years ago, machines resoundingly bested humans in International Chess when IBM’s Deep Blue Chess computer knocked Garry Kasparov off the reigning world Chess championship. However, artificial intelligence experts all thought at the time it would take many more years before computers would be able to beat humans in the game of Go because the game’s complex rules are thousands of times more complex. The Go man-machine contest is all the more an important milestone in the field of artificial intelligence and will certainly have ramifications for other fields such as natural language processing and translation. But compared to the black and white stone pieces of Go, mastering languages – with their large vocabularies and complex grammar rules and exceptions – is once again exponentially more difficult.
Today artificial intelligence is able to rival humans in many areas such as computer visual analysis in image classification (or object recognition), speech recognition and biological information management or bioinformatics. The next important milestone will be natural language processing, i.e. a better understanding of the written words and applying that knowledge to provide more accurate language translation.
Machine translation is here and now
AlphaGo’s victory against humans sends a strong rebuttal to MT (machine translation) doubters. The day for computers to perform some of the human tasks in the art of translation is around the horizon. Advancements in deep machine learning and neural networks allow computers to analyze increasingly complex information and then apply the computer’s superfast processing capabilities on that information to beat their human opponents. Compared to machines, the human brain is relatively weak in terms of memorizing information and much slower with calculations. Coincidentally, both of these capabilities are important for natural language learning. Furthermore, the machine’s ability to inherit 100% of the knowledge from previous generations effortlessly makes them a formidable contender for quality translation of the future.
Here are some more in-depth look at several major advantages of language learning by machines over their human counterparts:
- Machines are great at memorization. The inherent advantage of the computer is that it’s is able to remember sentences or text segments it has successfully processed in the past, and then performs grammatical processing to construct new sentences for performing natural language translation. Although this learning process is gradual, once mastered, the machine will be able to consistently translate content with sufficiently good accuracy at fast speed and low cost.
- Machines can perform calculations much faster than the human brain. The fact that we take much time to do simple math calculations is a result of the way human brains are wired to develop its reasoning. However, the human brain is by no means the only or best design in terms of fast calculations of complex equations. In fact, the computer has long been proved to be much superior to humans in churning out numbers of large and complex mathematical equations. This superior calculation ability gives machines huge advantage in terms of making sense of sentences it’s never seen before producing the best translations.
- Machines have total knowledge inheritance and knowledge transfer. One of the most frustrating human deficiencies is the inability for people to pass on the knowledge they have worked industriously to acquire to the next generation. The major “flaw” of human brain construction is that when someone dies, she or he takes away all that knowledge with them. This means each generation of humans have to spend a better part of their life recording their knowledge and the next relearning the knowledge their parents already learned. Therefore, the collective human knowledge development process is inefficient. When it comes to translation, each person has to learn a second from scratch. The sad thing is that when a good translator dies, all that great language knowledge is also gone, leaving little for the next translator to benefit. This is where machines excel, as the collection of knowledge is completely captured and saved in a central database for other machine processes to immediately duplicate and apply in their own translation work.
Machine translation will only get better as time goes on. However, does MT spell doom for the human translation effort? The answer is resoundingly no, at least not in our lifetimes.
One of the major benefits going for human translation is that language continues to evolve as a result of human endeavors. Specifically, new products, novel business concepts are introduced all the time and increasingly at fast speeds. This means new expressions and words and memes are invented by humans all the time to describe about brand new things. This also means humans will continue to stay head of the curve as far as language translation is concerned. Secondly, although machines are good at algorithmic analysis, they lack the appreciation of culture and context nuances that can only be mastered by a human translator. For transcreation, the combined activity of translating and rewriting for highly visible marketing content, machine translation is nowhere comparable to human translation. As a recent article on Japan Times pointed out, machine translation still can’t match a native’s touch.
In some ways, the MT effect is not all that different than what the word processor did for writing on a computer; they both make humans work more efficiently. In fact, machine translation is already widely used in today’s localization industry. More and more companies demand their localization service provider (LSP) incorporate MT in their translation workflow to achieve more cost efficiency and faster turnaround. In an MT assisted translation model, the human translator first applies MT to the source text before performing what is called post-editing to ensure grammatical accuracy and readability. The edited sentence is then stored in a database called TM (translation memory) for future translations; the process repeats itself so the machine learning process continuously generate better and better results in translation leverage, saving the customer both money and time.
Productivity gains in translation also make localization service less expensive. MT reduces the per word cost by allowing the translator to translate more words per day. Lowered translation costs allow companies to translate more content which business would otherwise not be translating due to higher costs. Most importantly, affordable translation services will encourage more companies to translate, increasing the demand for translation. Therefore the fear that machine translation will take over human translators’ jobs is overblown.
Translation service providers that take advantage of machine translation by incorporating MT into their translation model will be able to capture the most substantial market share. At the same time, translation technology that integrates machine translation into its localization workflow will prove to be the norm rather than the exception going forward. Better yet, next generation translation technology such as Stepes that are able to combine the power of machines, mobility and the language skills of human translators in a big translation approach will prove to be future winners.